Understanding Data Labeling in Machine Learning
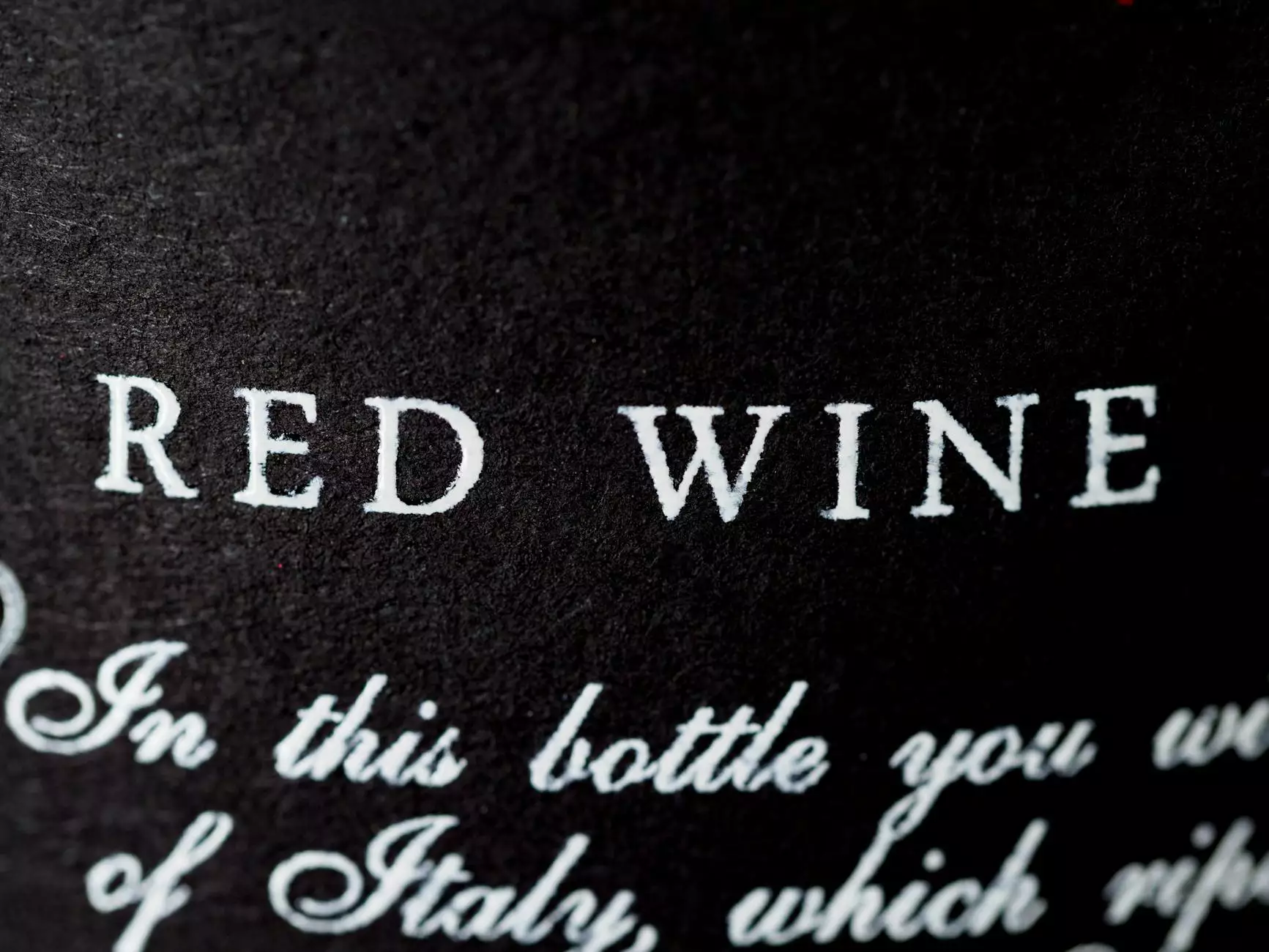
In today’s technology-driven world, data labeling has emerged as a fundamental component in the field of machine learning. Data labeling is the bridge that connects raw data to workable machine learning algorithms. This article will delve deeper into the concept of data labeling, its significance in machine learning, and how it benefits various industries, including Home Services and Keys & Locksmiths.
What is Data Labeling?
Data labeling refers to the process of tagging or annotating raw data to make it understandable and usable for machine learning algorithms. It is the foundation upon which machine learning models are built. The primary aim of data labeling is to train algorithms to recognize patterns, make predictions, and achieve high performance when processing real-world data.
The Importance of Data Labeling in Machine Learning
Data labeling plays a vital role in ensuring the success of machine learning models. Here are several reasons why:
- High-Quality Output: Machine learning models learn from labeled data. The more accurately data is labeled, the better the model’s performance and predictions.
- Improved Accuracy: Labeled data helps to reduce errors in predictions, leading to improved accuracy in tasks like image recognition, text classification, and more.
- Customized Solutions: By labeling data specific to your domain, you can create customized solutions tailored to your business needs.
Types of Data Used in Machine Learning
Data labeling can be applied to various types of data, including:
1. Image Data
In image data labeling, images are annotated with bounding boxes, polygons, or keypoints to identify objects or features within the images. This is particularly useful in areas such as security systems and home automation.
2. Text Data
Text data labeling involves categorizing or tagging text documents for tasks such as sentiment analysis or spam detection. For locksmith businesses, this can include classifying customer inquiries or reviews to better understand client needs.
3. Audio Data
In audio data labeling, sound clips are annotated to identify speech, background noise, or sound events. This can be crucial for voice-activated security systems integrated into home services.
The Data Labeling Process
The data labeling process typically involves several stages:
1. Data Collection
Gather raw data from various sources relevant to the business, ensuring it is representative and comprehensive.
2. Data Annotation
Utilize labeling tools and techniques to annotate the collected data accurately. Techniques can include manual labeling or automated labeling supported by AI.
3. Quality Assurance
Implement quality checks to ensure that the labeled data is accurate and meets the required standards. This step is critical to eliminate errors that could affect model performance.
Tools for Data Labeling
There are several tools available for data labeling, and choosing the right one depends on your specific requirements:
- Labelbox: An intuitive platform that allows teams to collaborate on labeling projects.
- Amazon SageMaker Ground Truth: This tool offers a set of built-in workflows for different types of data, enhancing efficiency.
- SuperAnnotate: A comprehensive suite tailored for image and video annotation with collaboration features.
Challenges in Data Labeling
Despite its importance, data labeling comes with its own set of challenges:
1. Time Consumption
Labeling large datasets can be time-consuming and labor-intensive, leading to potential delays in project timelines.
2. Human Error
Manual labeling is prone to human error, which can adversely affect the performance of machine learning models.
3. High Costs
Outsourcing data labeling can be costly, especially for businesses with limited budgets. However, the benefits often outweigh the costs when done correctly.
Best Practices for Effective Data Labeling
Implementing some best practices can vastly improve your data labeling efforts:
- Define clear labeling guidelines: Establish specific definitions and examples to maintain consistency across the labeled dataset.
- Implement a review process: Regularly review labeled data for quality and accuracy, incorporating feedback for continuous improvement.
- Utilize semi-automated labeling: Leverage machine learning algorithms to assist with labeling, saving time while maintaining quality.
How Data Labeling Benefits the Home Services Sector
In the Home Services industry, data labeling can significantly enhance operational efficiency and customer satisfaction:
1. Improved Customer Interaction
By accurately labeling customer inquiries and feedback, businesses can tailor their responses and services to better meet client needs.
2. Enhanced Marketing Strategies
Data labeled for sentiment analysis can help identify customer trends and preferences, informing targeted marketing campaigns.
3. Operational Optimization
With labeled data regarding service requests, businesses can analyze patterns and optimize their field operations accordingly.
Data Labeling in the Locksmith Industry
For the Keys & Locksmiths business, data labeling holds immense potential:
1. Fraud Detection
By labeling historical service calls and customer data, locksmiths can identify potentially fraudulent activity, enhancing security measures.
2. Service Personalization
Labeling data on customer preferences and lock types allows locksmiths to provide personalized recommendations, improving service experience.
3. Predictive Maintenance
Data labeling aids in predicting maintenance needs by analyzing customer service records, ensuring proactive service delivery.
Future of Data Labeling in Machine Learning
The future of data labeling is poised for transformation, with advances in technology paving the way for innovative solutions:
- AI-Driven Tools: The integration of AI and machine learning in data labeling tools will enhance efficiency and minimize human errors.
- Crowdsourced Labeling: Platforms that leverage crowd-sourcing for data labeling can offer scalable solutions with diverse input.
- Automated Quality Checks: Future data labeling solutions will likely include automated checks to ensure high-quality output, reducing the resource burden on businesses.
Conclusion
In conclusion, data labeling is an indispensable part of machine learning that significantly enhances the capabilities of models across various industries, including Home Services and Keys & Locksmiths. By understanding the importance of data labeling and implementing best practices, businesses can harness the power of machine learning to drive growth, enhance customer satisfaction, and optimize operational efficiency. As technology continues to evolve, embracing advancements in data labeling will be crucial for staying ahead in the competitive landscape.
data labeling machine learning